Community | People
Online And Offline Retailing - Current State And Future Strategies
Sep 13, 2023 | K Bhuvan Sai
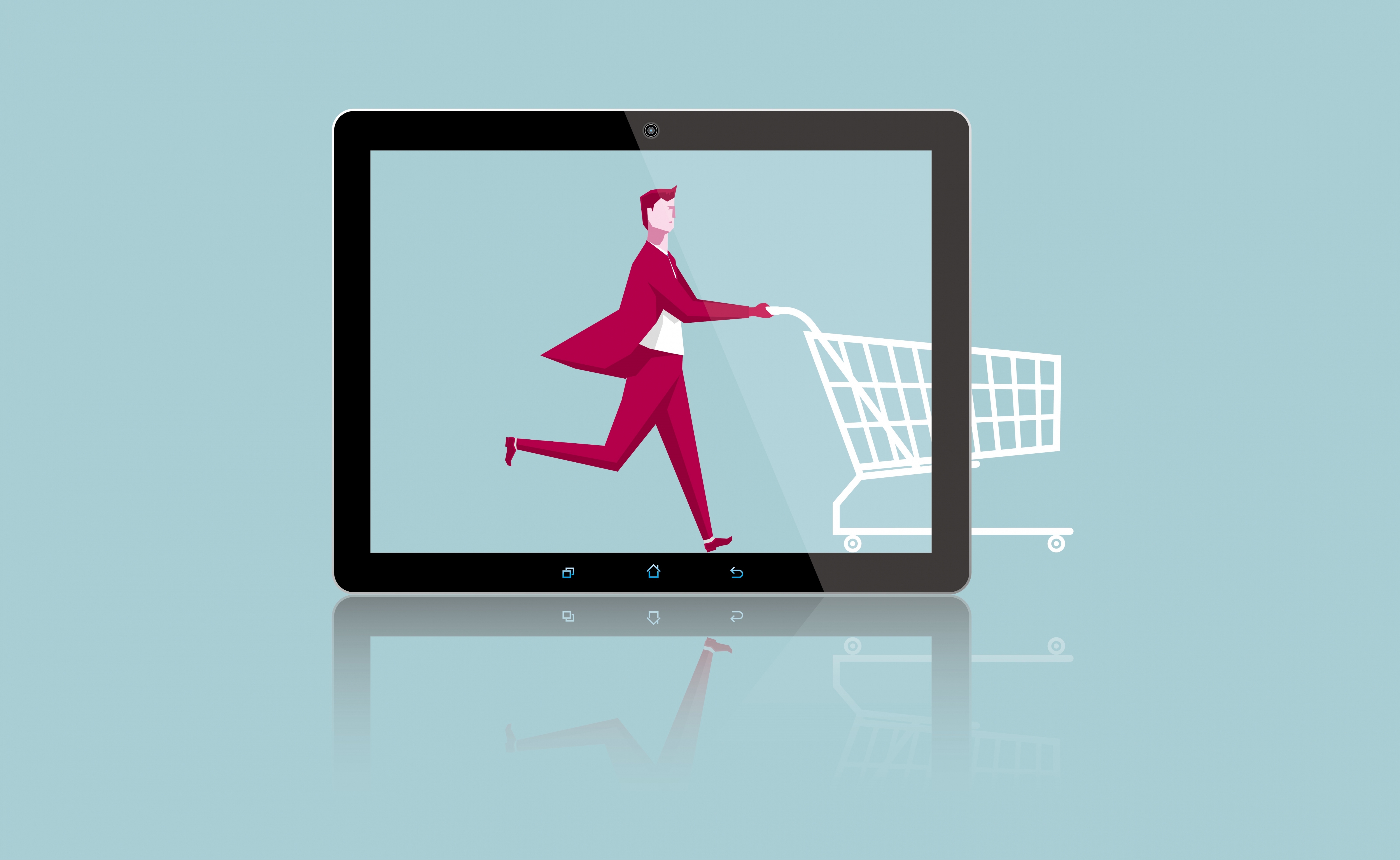
Online Retailing Trends and Statistics
The body of the report delves into the latest trends and statistics in the online retailing industry. It highlights the exponential growth of e-commerce, the increasing usage of mobile devices for shopping, and the emergence of new retail technologies.
Challenges Faced by Online Retailers
This section explores the challenges that online retailers encounter in an increasingly competitive market. Challenges such as inventory management, supply chain logistics, and customer retention are discussed.
Integration of Online and Offline Retailing
The report examines the concept of an omnichannel approach, wherein online and offline retailing channels are integrated seamlessly. It discusses the benefits of providing customers with options to shop online and pick up items in-store, as well as the use of data analytics to enhance personalization across both channels.
Checkout Optimization Techniques
The report outlines various checkout optimization techniques for online retailers. This includes streamlining the checkout flow, implementing user-friendly payment gateways, and ensuring a secure and trustworthy transaction process.
What is the impact of big data on retailing?
Big data is characterized by large volumes of data that require special storage facilities (volume), need for timely access (velocity), a variety of formats (variety), reliability, and validity (veracity) – (Wedel and Kannan, 2016). The term “big data” applies most directly to data that became available with the advent of the internet, which led to the availability of data on online purchases, clickstream data, online review data, data on individual responses to advertisements, data on the timing of browsing and purchases, data on consumers’ physical locations, data on social network participation, and data on competitors’ prices and online advertising (Bradlow et al., 2017). All of this is in addition to the existing point-of-sale data on offline purchases. Bradlow et al. (2017) and Wedel and Kannan (2016) provided extensive discussions of the use of big data in marketing, in general we will focus on the application of big data to marketing decisions in retailing, including customer relationship management (CRM), product, and marketing mix decisions.
Figures, Tables, Equations, and Formulae
In the closed-form expressions of retailer profits, we fix the values of base utility, mismatch cost, and mismatch cost scaling parameter so that we can analyze the equilibrium in terms of the proportion of CC consumers and revenues from targeted advertising. Our analysis of the conditions in which a symmetric or asymmetric equilibrium holds reveals two symmetric equilibria: one when both retailers use a restricted strategy and another in which both retailers use a flexible strategy and one asymmetric equilibrium when retailer 1 adopts a flexible checkout strategy, and retailer 2 adopts restricted checkout strategy, as we detail in Proposition 1 and 2.
Proposition 1. The asymmetric equilibrium with one retailer choosing the restricted checkout strategy and the competing retailer choosing a flexible checkout strategy holds when the proportion of CC consumers is relatively high (α > α1 ) and revenues due to targeted advertising are in the intermediate range (η1 < η < η2 ).
The result is driven by three key factors that operate in our modeling framework: (i) additional revenues from advertising (sharing of information about registered customers with advertisers could increase profits, (ii) utility due to account registration since a higher allows firms to charge rice’s, (iii) proportion the two types consumers market it impacts total demand obtained retile under each strategy. When offers a flexible checkout strategy, both purchase retailer. Consider a scenario in which revenue targeted parameter intermediate range, and retailers are following As CC increases, one realizes prices catering only foregoing PC consumers. prefer to buy the guest option. So, they will strategy.
Alternately, consider the scenario in which the proportion of CC consumers is high, and advertising in revenue parameter is relatively low. As parameter increases, additional from as well ability to charge higher prices, incentivize retailers to switch to a flexible checkout strategy restricted ven though restricted eliminating demand for PC consumers. The competing retailer continues to able to generate profits also. Thus, an asymmetric could arise equilibrium. By considering two distinct consumer types, our result provides a demand-based (rather than just cost-based) justification and plausible explanation for why horizontally differentiated may adopt an equilibrium.
Proposition 2. (a) The symmetric equilibrium with both retailers choosing a restricted checkout strategy holds when the proportion of CC consumers and the targeted advertising revenue parameter are relatively high (α > α2 and η > η2 ). (b) The symmetric equilibrium with both retailers offering a flexible checkout strategy always holds when the targeted advertising revenue parameter is below a given threshold (η < η1 )
The intuition for this result is as follows: When the proportion of CC consumers is relatively lower, it implies that choosing a restricted checkout strategy ignores a large portion market. Further, when coupled with revenues from targeted advertising being offered flexibly enables online retailers to generate additional demand for PC consumers. Thus, we observe flexible strategies in equilibrium proportion and revenue parameters are lower. In contrast, both these parameters exceed given thresholds, and profits serving only exceed incremental generation choose use equilibrium. Fig. 1, shows regions Case 1. As mentioned previously, about 35% of users cite the account creation process key reason for abandoning implying α = 0.65. We 0.65, FF, FR, RR could exist equilibrium depending on values other (as shown 1). Our results broadly consistent notion competing practice may be any combination of two η (additional advertising) parameter changes. order to understand the above propositions further, calculate comparative statistics retailers’ respect some underlying model analysis presented in Proposition 3.
Proposition 3. (a) Under the symmetric R-R or the asymmetric equilibriums, firm profits always increase with the increase in the proportion of CC consumers dπ∗ iRR dα > 0 and dπ∗ iFR dα > 0, whereas, under the symmetric F-F equilibrium, firm profits decrease with increase in the proportion of the CC consumers dπ∗ iFF dα < 0 for α < α3. (b) Under the symmetric R-R equilibrium, firm profits are invariant to increase in the additional revenues due to targeted advertising parameter dπ∗ iRR dη = 0. In contrast, under the symmetric F-F equilibrium, equilibrium profits decrease with an increase in the additional revenue due to targeted advertising dπ∗ iFF dη < 0. Further, under the asymmetric equilibrium, the profits of the retailer that adopts a restricted strategy always increase with the increase of additional targeted advertising revenue parameter π∗ 2FR dη > 0 whereas the profit of the retailer that adopts a flexible strategy always decreases with the increase in the additional revenues due to targeted advertising parameter when the additional revenues due to targeting exceed a threshold dπ∗ 1FR dη < 0 for η > η3.
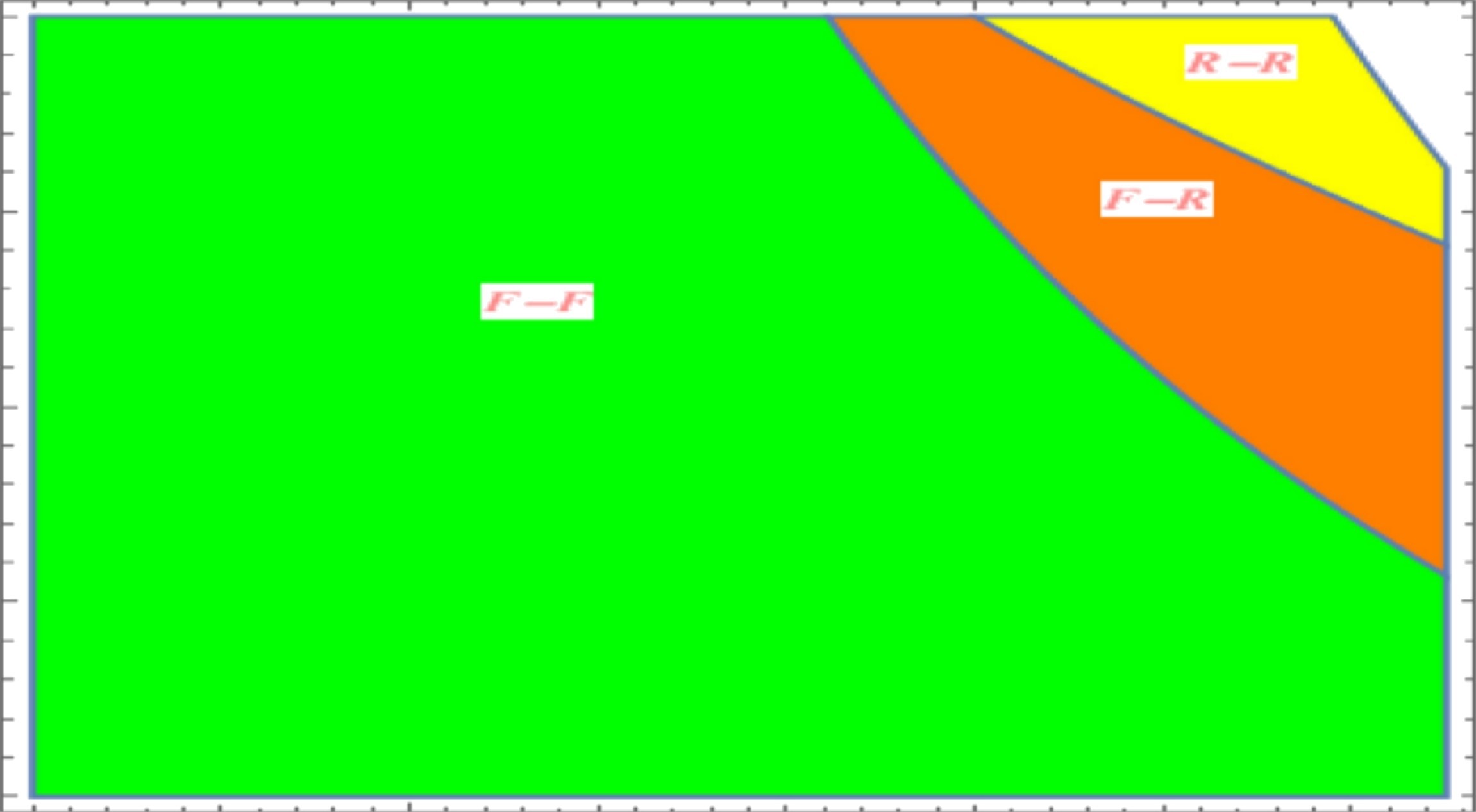
Proposition 4. The asymmetric equilibrium, with one retailer utilizing a restricted checkout strategy and the competing retailer utilizing a flexible checkout strategy, holds under two conditions: (i) when the proportion of CC consumers as well as the mismatch cost scaling parameters are in the intermediate range (α4 < α < α5 and θ1 < θ < θ2 ), OR (ii) when the proportion of CC consumers as well as mismatch cost scaling parameter are relatively high (α > α6 and θ > θ3 ).
Proposition 5. (a) The symmetric equilibrium with both retailers follows a restricted checkout strategy holds when the proportion of CC consumers is in the intermediate range (α7 < α < α8 ) and the mismatch cost scaling parameter is relatively low (θ < θ4 ). (b) The symmetric equilibrium with both retailers following a flexible checkout strategy always holds when the proportion
of CC consumers is below a threshold (α ≤ α9 ).
Proposition 6. (a) Under the symmetric R-R and F-F equilibria, firm profits always increase with
increase in the mismatch cost scaling parameter dπiFF dθ > 0 and dπiFF dθ > 0. (b) Under the asymmetric equilibrium, the profits of the retailer that adopts a flexible checkout strategy always increase with an increase in mismatch cost scaling parameter dπ1FR dθ > 0 whereas the profits of the retailer which adopts a restricted checkout strategy increases with increase in mismatch cost scaling parameter only when the proportion of CC consumers exceeds a threshold dπ2FR dθ > 0 for α.
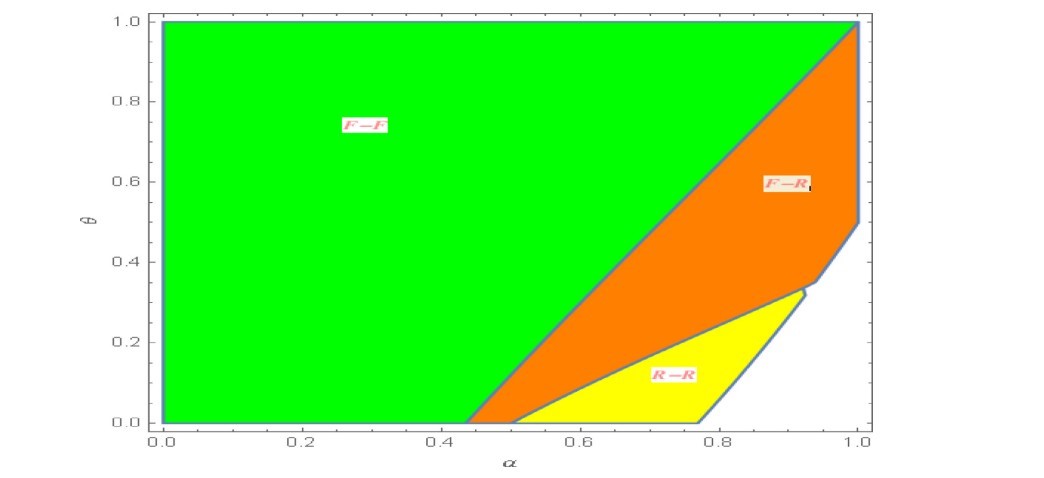
Conclusion
In conclusion, the technical report presents a comprehensive analysis of the online and offline retailing industry, including its current state, challenges, and future research directions. It emphasizes the importance of optimizing the online checkout process to enhance customer satisfaction and boost conversion rates.
Recommended
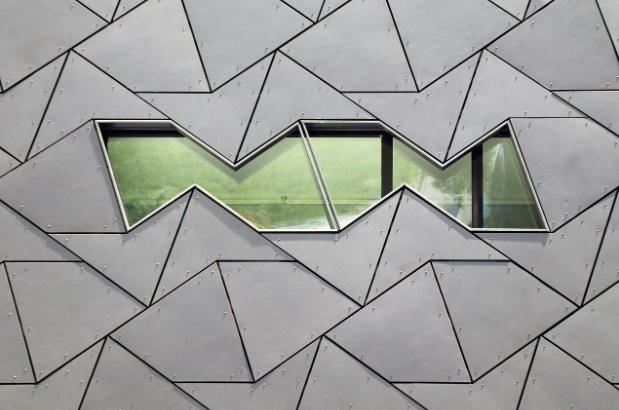
Business Management | Resources
In 2021, Rae Dunn’s transformation from a small-scale ceramic artist selling at craft fairs to a multi-million-dollar brand showcased the extraordinary potential of handmade businesses. What started as a passion for simple, hand-lettered ceramics evolved into a coveted collection, with fans lining up at stores before sunrise. Beyond the clay and glaze, her success hinged on a unique brand identity, unwavering customer loyalty, and demand-driven pricing.
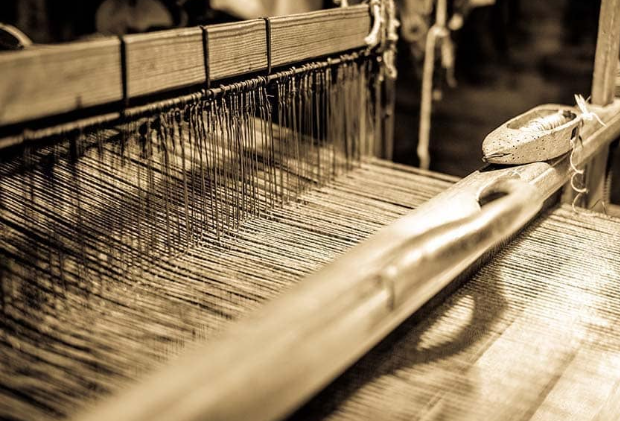
Business Management | Resources
In 2021, Rae Dunn’s transformation from a small-scale ceramic artist selling at craft fairs to a multi-million-dollar brand showcased the extraordinary potential of handmade businesses. What started as a passion for simple, hand-lettered ceramics evolved into a coveted collection, with fans lining up at stores before sunrise. Beyond the clay and glaze, her success hinged on a unique brand identity, unwavering customer loyalty, and demand-driven pricing.
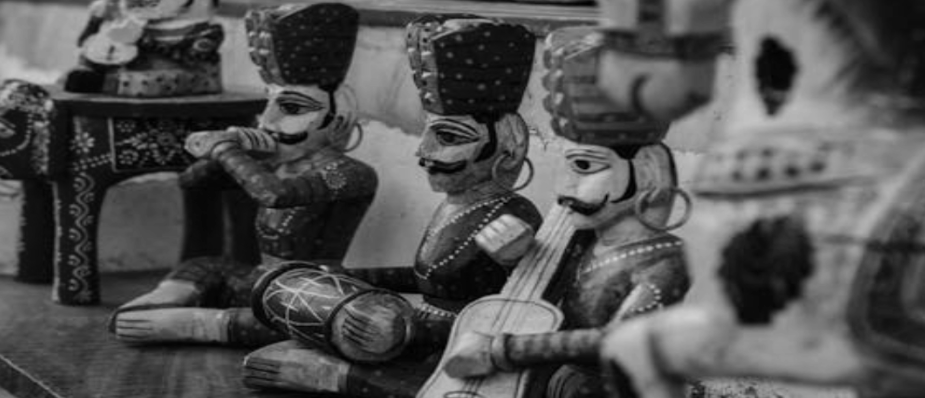
Business Management | Resources
In 2021, Rae Dunn’s transformation from a small-scale ceramic artist selling at craft fairs to a multi-million-dollar brand showcased the extraordinary potential of handmade businesses. What started as a passion for simple, hand-lettered ceramics evolved into a coveted collection, with fans lining up at stores before sunrise. Beyond the clay and glaze, her success hinged on a unique brand identity, unwavering customer loyalty, and demand-driven pricing.