Evaluating Strategies For Promoting Retail Mobile Channel Using A Hidden Markov Model
Sep 10, 2023 | Varun Gopi
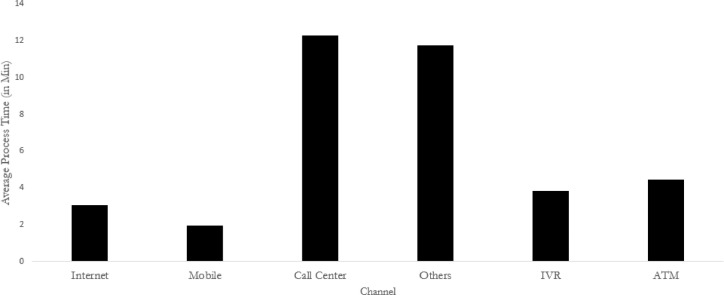
Understanding Hidden Markov
Model (HMM):
A Hidden Markov Model is a statistical model used to represent
systems with hidden states that
generate observable outcomes. In the context of retail, the HMM can help
uncover latent customer behavior
states, which influence their interactions with the mobile channel. These hidden
states could include "engaged," "disengaged," or "inactive" customer
phases.
Data Collection and Preprocessing:
To implement the HMM, retailers need to gather relevant data from their mobile applications and websites. This data might include customer interactions, click-through rates, purchase history, session durations, and demographic information. Once collected, the data undergoes preprocessing, where it is cleansed, transformed, and structured for analysis.
Model Training and State Estimation:
The next step involves training the Hidden Markov Model using the preprocessed data. During the training process, the model learns the underlying transition probabilities between different hidden states, based on observed customer behavior patterns. Once trained, the HMM can then be used to estimate the most likely hidden state sequences for new customer interactions.
Identifying Effective Promotion Strategies:
By leveraging the HMM, retailers can identify which promotion
strategies lead to higher engagement
and conversion rates. The model can help determine which customer segments respond
best to specific promotions, tailoring marketing efforts accordingly. For instance, the HMM
might reveal that offering time-sensitive discounts to a particular segment
leads to increased mobile app usage and purchases.
Personalization and Customer Experience:
A crucial advantage of using an HMM is its ability to facilitate
personalized customer experiences. By
understanding individual customer behavior patterns, retailers can deliver targeted
promotions, product recommendations, and marketing messages
that align with each customer's preferences. This
personalization can significantly enhance customer satisfaction and loyalty.
A/B Testing and Model Refinement:
To continuously improve the mobile channel promotion strategies,
retailers can implement A/B testing.
By comparing the performance of different strategies, they can validate the HMM's insights and refine their
models accordingly. This iterative approach
helps optimize marketing efforts for ongoing
success.
Limitations and Future Directions:
While the HMM is a powerful tool, it does have some limitations.
For instance, the accuracy of the model heavily depends on the
quality and quantity of the input data. Additionally, the HMM assumes that customer behavior adheres
to Markovian properties, which might not always
hold true in practice. Future research could focus on combining HMM with other advanced
techniques to address
these limitations.
Conclusion:
Using a Hidden Markov Model to evaluate and promote the retail
mobile channel is a data-driven approach that can yield valuable insights and enhance customer engagement. By understanding hidden customer behavior
states, retailers can tailor their promotional
strategies and create
personalized experiences that drive conversions and foster brand loyalty. As the retail industry continues to
evolve, leveraging advanced analytical models like the HMM will play an increasingly vital role in staying competitive
and relevant in the mobile-driven landscape.
Recommended
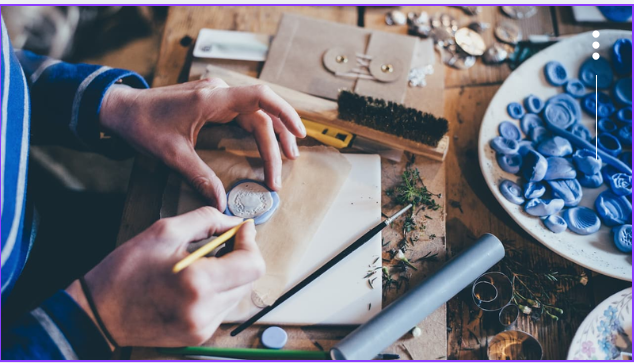
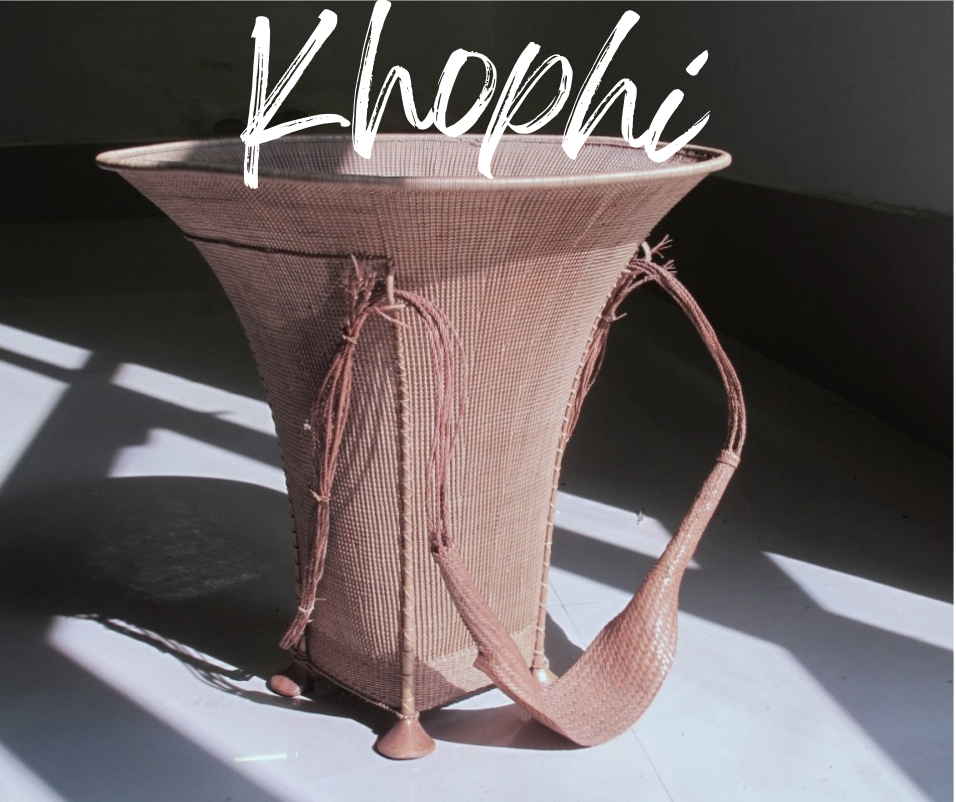
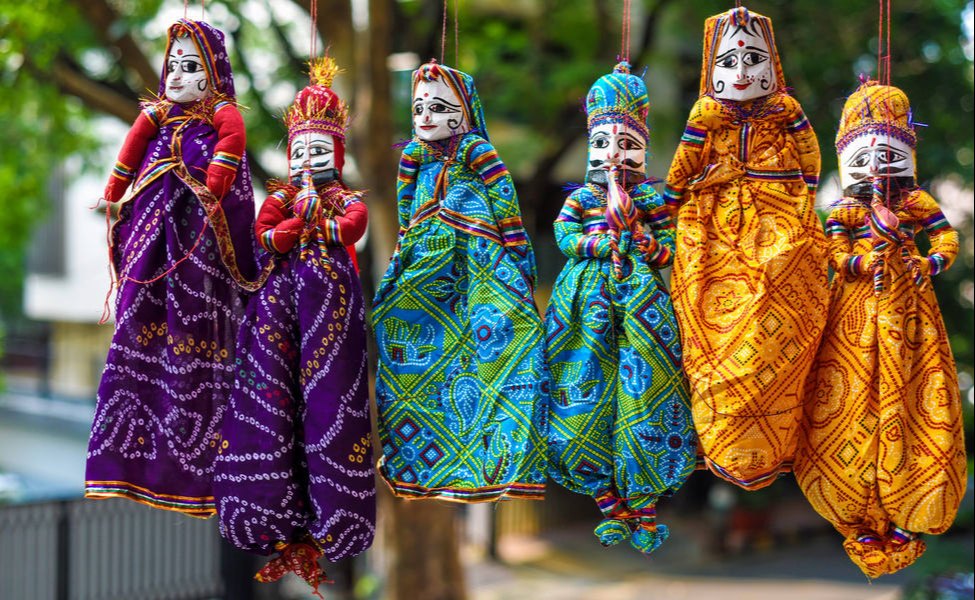
Wholesale / B2b Kathputli Puppets From Ahemdabad
Dec 16, 2024