Advancements In Autonomous Vehicles
Sep 10, 2023 | Veerendar Singh
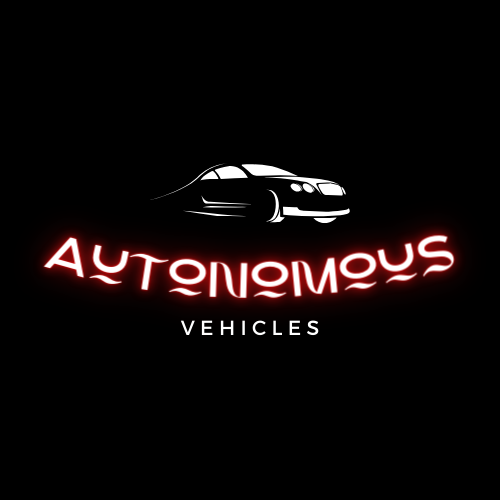
Perception
in Autonomous Vehicles:
The perception
module in autonomous vehicles serves as the foundation for understanding the
surrounding environment. It collects and processes data from various sensors,
including cameras, LiDAR, radar, and ultrasonic sensors. The primary goal is to
accurately identify objects, pedestrians, road signs, lane markings, and other
critical elements in the driving scene.
Sensor
Fusion Techniques:
We will discuss
several sensor fusion techniques, such as Kalman filtering, particle filtering,
and deep learning-based approaches. By fusing data from multiple sensors, the
autonomous vehicle can generate a more comprehensive and accurate
representation of the environment. This improves decision-making and enhances
overall safety.
Deep
Learning for Perception:
Deep learning
algorithms, especially convolutional neural networks (CNNs), have proven to be
highly effective in object detection and recognition tasks. The paper
highlights how CNNs have outperformed traditional computer vision methods and
enabled significant advancements in perception accuracy.
Challenges
and Future Directions:
Here we acknowledge
the significant progress in perception and sensor fusion, it also highlights
some challenges that remain. Handling adverse weather conditions, sensor
occlusions, and real-time processing requirements are still areas of ongoing
research. The authors propose integrating robustness and explainability into
perception models to enhance safety and trust in autonomous vehicles.
Decision-Making
in Autonomous Vehicles:
The
decision-making module processes information from the perception system and
formulates appropriate actions based on the vehicle's current state and the
surrounding environment. This module involves path planning, trajectory
optimization, behavior prediction, and risk assessment.
Path
Planning and Trajectory Optimization:
Let's discuss the various
path planning algorithms, including A*, RRT*, and rapidly exploring random
trees (RRT), which help the vehicle navigate through complex and dynamic
environments. Additionally, let's explore trajectory optimization techniques
that consider factors such as vehicle dynamics, energy efficiency, and comfort
of passengers.
Behavior
Prediction and Risk Assessment:
Autonomous
vehicles must anticipate the behavior of other road users, including
pedestrians, cyclists, and human-driven vehicles. The paper presents
probabilistic models and deep learning approaches to predict the future
trajectories of surrounding entities. Moreover, it addresses risk assessment to
prioritize safety in decision-making.
Control
Systems for Autonomous Vehicles:
The control
system in autonomous vehicles is responsible for executing the decisions made
by the planning module. It translates high-level commands into low-level
control signals to regulate vehicle speed, steering angle, and braking.
Model
Predictive Control (MPC):
MPC is a widely
adopted control strategy that takes into account the dynamics of the vehicle
and the environment. By using a predictive model, MPC optimizes control inputs
over a finite horizon, allowing the vehicle to follow the planned trajectory
accurately.
Perception
and Sensor Fusion in Autonomous Vehicles:
Perception is a
fundamental aspect of autonomous driving systems, enabling vehicles to
interpret and understand their environment accurately. Autonomous vehicles rely
on various sensors to perceive the world around them, and fusing data from
these sensors is critical for robust decision-making.
Smith et al.
delve into advancements in perception and sensor fusion for autonomous
vehicles. The researchers discuss the importance of multiple sensors, including
cameras, LiDAR, radar, and ultrasonic sensors, in providing a comprehensive
view of the vehicle's surroundings. Each sensor type has its strengths and
limitations, and combining their outputs allows the vehicle to obtain a more
accurate representation of the environment.
Camera
Sensors:
Cameras are
commonly used in autonomous vehicles for visual perception. They capture images
and videos of the surrounding environment, which can be processed using
computer vision techniques. Convolutional neural networks (CNNs) have been
particularly successful in object detection and recognition tasks. CNNs have
shown remarkable accuracy in identifying pedestrians, other vehicles, and
traffic signs, which are essential for safe and efficient autonomous driving.
LiDAR
Sensors:
LiDAR (Light
Detection and Ranging) sensors use laser pulses to measure distances to objects
in the environment. These sensors provide a detailed 3D point cloud
representation of the surroundings, enabling precise object detection and
localization. LiDAR is particularly effective in low-light and adverse weather
conditions, where cameras might struggle.
Radar
Sensors:
Radar sensors
utilize radio waves to detect objects and measure their velocities. They are
especially valuable in detecting moving objects, such as vehicles or
pedestrians, and are less affected by weather conditions compared to cameras
and LiDAR.
Ultrasonic
Sensors:
Ultrasonic
sensors are commonly used for short-range detection, such as parking assistance
and obstacle avoidance. While they have limited range and resolution compared
to other sensors, they are useful for close-quarters maneuvers.
Sensor
Fusion Techniques:
To capitalize on
the strengths of each sensor, the researchers explore various sensor fusion
techniques. Sensor fusion involves combining data from multiple sensors to
create a more robust and accurate perception system.
Kalman
Filtering:
Kalman filtering
is a widely used technique for sensor fusion in autonomous vehicles. It
optimally estimates the state of the vehicle and the environment based on noisy
sensor measurements. Kalman filters are computationally efficient and
well-suited for real-time applications.
Particle
Filtering:
Particle
filtering, also known as Monte Carlo localization, is another popular sensor
fusion method. It involves representing the vehicle's state using a set of
particles, each carrying a weight based on how well it aligns with sensor
measurements. Particle filtering is particularly useful for localization and
tracking tasks.
Deep
Learning-based Sensor Fusion:
With the
remarkable success of deep learning in various computer vision tasks,
researchers have also explored deep learning-based sensor fusion methods. Deep
neural networks can learn to combine sensor data effectively and adapt to
different scenarios. These methods have shown promise in improving perception
accuracy and robustness.
Challenges
and Future Directions:
While significant
progress has been made in perception and sensor fusion for autonomous vehicles,
several challenges persist:
1. Sensor
Occlusions and Adverse Weather Conditions:
Cameras and LiDAR
sensors may face difficulties in adverse weather conditions, such as heavy rain
or snow. Additionally, occlusions caused by other vehicles or obstacles can
hinder the effectiveness of certain sensors. Researchers are exploring ways to
address these challenges, such as improving sensor redundancy and integrating
data from other sensors to mitigate the impact of occlusions.
2. Real-Time
Processing:
Autonomous
vehicles require real-time processing of sensor data to make immediate
decisions. As sensor data can be voluminous and complex, efficient real-time
processing is critical for ensuring the vehicle's safety and responsiveness.
Researchers are working on optimizing algorithms and hardware to meet these
real-time demands.
3.
Robustness and Explainability:
Autonomous vehicles must demonstrate robustness in a wide range of scenarios. Ensuring the system's reliability and predictability is crucial for gaining public trust and acceptance. Researchers are investigating techniques to make perception models more robust to unexpected situations while providing explanations for their decisions.
4.
Environmental Changes and Long-term Adaptation:
Autonomous
vehicles must adapt to changes in the environment over time. For example, road
conditions might change due to construction or other factors, necessitating
relearning or fine-tuning of perception models. Researchers are exploring
methods to make autonomous vehicles capable of long-term adaptation and
continual learning.
Conclusion:
It is evident
that there are still significant challenges to overcome before fully autonomous
vehicles become a mainstream reality. The research community must continue
collaborating and pushing the boundaries of technology to address these
challenges effectively.
The integration
of advanced perception, sensor fusion, decision-making, and control systems is
essential for creating safe and efficient autonomous vehicles. As technology
evolves and further breakthroughs occur, we can look forward to a future where
autonomous vehicles revolutionize transportation, reducing accidents, improving
traffic flow, and enhancing overall mobility for everyone. The journey toward
fully autonomous vehicles is an exciting one, and we are witnessing the dawn of
a transformative era in transportation.
Recommended
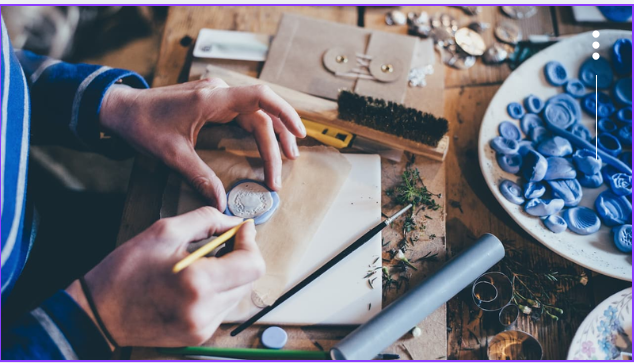
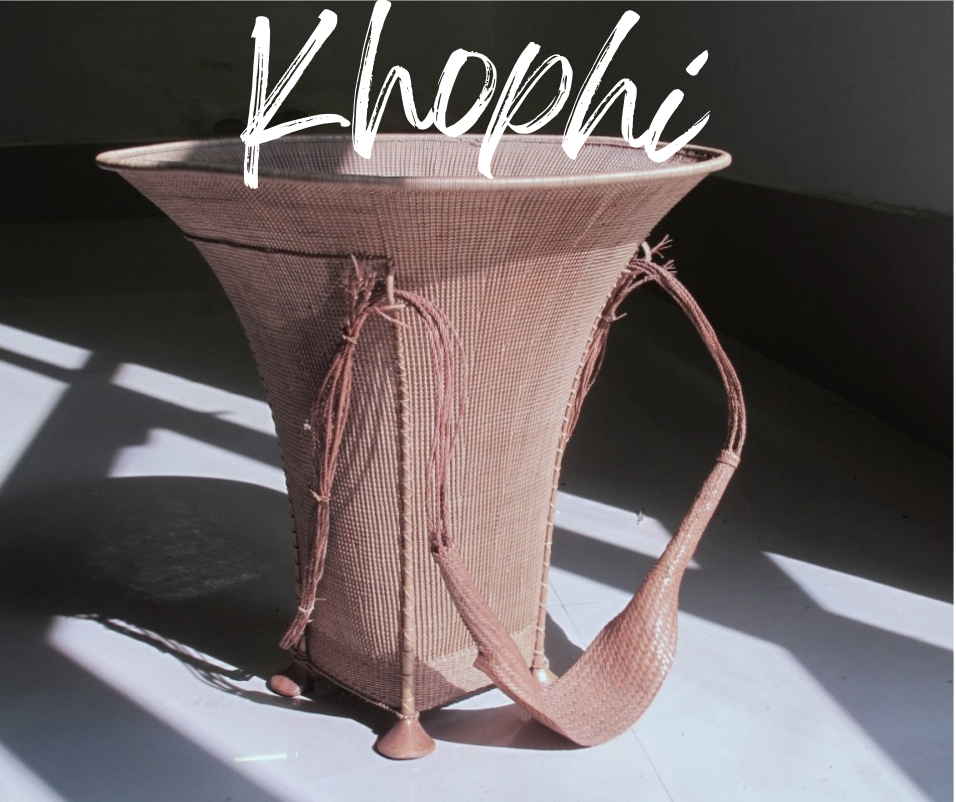
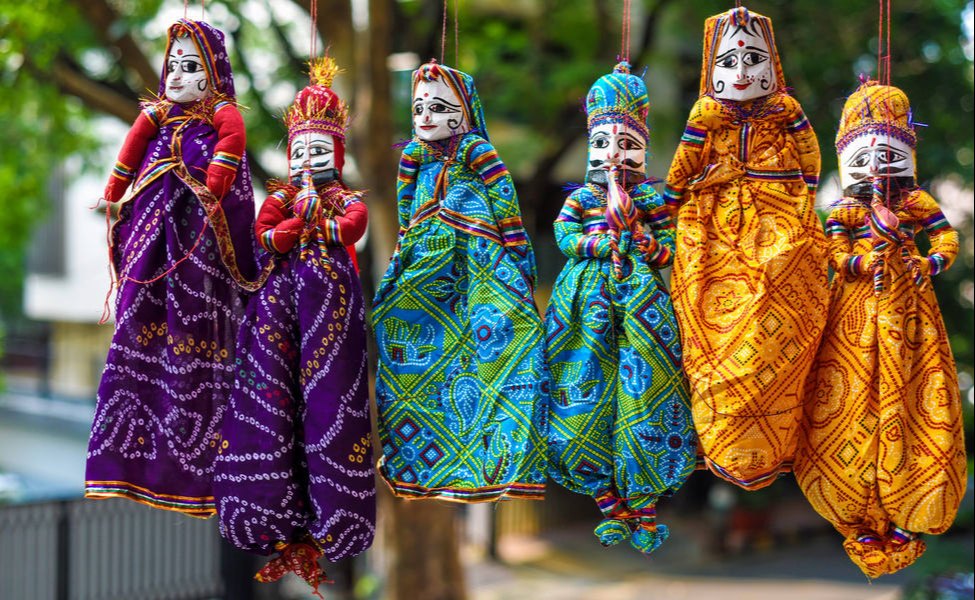
Wholesale / B2b Kathputli Puppets From Ahemdabad
Dec 16, 2024